How generative AI can improve your knowledge management
February 21 · 05 mins read
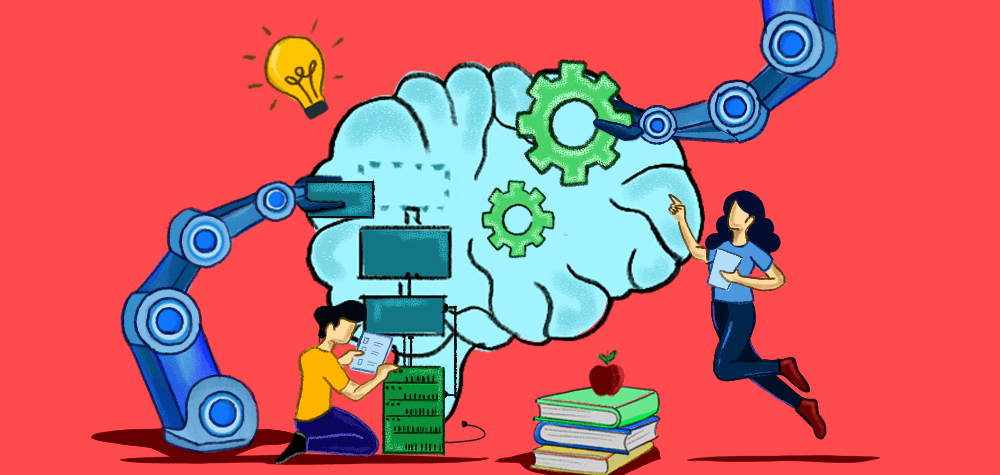
We have all been there—when faced with a problem or an urgent request, we turn to the knowledge base for help. Too often, we scour through an endless pool of documentation, only to find out that nothing matches our predicament. Like always, the inevitable ticket to the service desk becomes our last resort, leaving us to play the waiting game until the IT team responds. All of this could have been avoided had there been easy access to the relevant solutions up front.
An organized knowledge management strategy, therefore, can be the difference between swift issue resolution and prolonged downtime. However, despite its clear benefits, many organizations still seem to grapple with suboptimal outcomes. According to The AXELOS ITSM Benchmarking Report 2022, knowledge management is the sixth-most adopted ITIL® practice but only has a self-reported success level of 20%.
One major reason is that traditional knowledge management systems are often static and one-dimensional in the sense that they rely on manual curation and updating of information. Also, knowledge is a dynamic entity that requires constant evolution to maintain its relevance.
Thankfully, recent technological advancements have helped address these gaps by positioning knowledge management to transition from an administrative activity of documenting and sharing information to a more interactive, intelligent approach.
Powered by AI and machine learning, these advancements, especially in the form of generative AI tools, can help organizations generate and disseminate knowledge with greater efficiency than ever before.
Is generative AI only for tech giants?
Businesses are now starting to understand how generative AI can be harnessed to tackle the difficulties of collecting, organizing, and transferring knowledge across the enterprise. Large language models (LLMs) have brought a wave of innovation to tasks like generating text and understanding language. However, their large scale and computational demands have limited their adoption to only organizations with extensive financial and technological capabilities.
While publicly available LLMs such as GPT-4 and PaLM 2 demonstrate impressive performance, simply applying these models to enterprise use cases can pose certain challenges for corporate use. Also, smaller organizations may not have the resources to leverage them.
Below are some key areas where organizations find themselves in the lurch with generic models:
A lack of domain-specific data and context | General purpose models respond effectively to generic questions but not as well when queried about specific products or services. This knowledge gap makes the models prone to hallucinations and biases. |
Resource-intensive training and maintenance | Training and maintaining LLMs involves substantial resources and computational requirements, making LLMs exclusive to well-funded organizations. |
A lack of data security | Concerns surround the security and privacy of organizations' proprietary data. Organizations would rather have this data within their control than allow a public-facing LLM to train on it. |
For enterprises to reap the full benefits of generative AI in their knowledge management strategy, they must overcome these challenges first. Recently, the realization dawned that the way forward to tap into the true potential of generative AI is to pivot towards smaller, more domain-specific models. These small language models, by being trained on the organization's corpus of data, can offer capabilities similar to their larger counterparts but in a more resource-efficient manner. This will make generative AI more accessible to a broader audience.
Here are some factors driving the development of smaller language models:
Enhanced customization | Small language models, when trained on domain-specific use cases and internal enterprise data, provide a higher degree of customization tailored to the organization's unique needs. |
Manageable training and deployment | The training and deployment of these models is more manageable, requiring far less training data and less powerful hardware. This results in substantial cost savings for the organization in the long run. |
Data control | Training the model on curated datasets within a controlled environment helps protect sensitive information and maintain privacy, reducing the risk of unauthorized access to enterprise data. |
The emergence of small language models marks a pivotal moment in AI, helping us unlock a myriad of uses in enterprise knowledge management. Let's then explore how generative AI can help service desks address the inherent challenges of sharing knowledge across the enterprise.
3 ways service desk teams can optimize their knowledge management with generative AI
1. Knowledge creation: Enrich your knowledge base by generating detailed solution articles
Converting implicit knowledge to explicit knowledge is the key to successful knowledge management. That conversion requires detailed input from various technicians and subject matter experts. With their plates already full, they may not always have time to record their resolution process and write solution articles for the knowledge base.
Thankfully, with generative AI capabilities, technicians can accelerate the writing process by converting various pieces of information, such as data found in a ticket's notes, attachments, or work logs, into comprehensive articles. Based on prior training on ticket data and other solution articles, language models can extract information from previously captured knowledge. That information is then used to generate detailed solution articles.
This simplifies the writing process for technicians, as reviewing and revising this generated solution is much easier than drafting one from scratch. Creating intricate knowledge documents for different kinds of tickets can help service desks enhance their knowledge base over time.
2. Knowledge discovery: Improve knowledge search by filling the semantic gap
To maximize your knowledge base, you need to optimize knowledge discovery first. Every moment saved in the process of knowledge discovery is a win, considering that the average employee spends about 3.6 hours per day searching for information.
The semantic gap, which is the divide between what users intend to find and the search results they receive, has always been a significant drawback of static knowledge management. Traditional systems that rely on rigid keyword overlap often fall short in comprehending user intent and specific context.
That is why it is important to have a knowledge management system that offers an intuitive search function to help users find context-specific answers. With the advanced natural language processing capabilities of generative AI, matching a searcher's intent to relevant responses has become possible.
Since language models are designed to understand the subtle nuances of human language, they can handle various user inputs, regardless of how the questions are phrased. As a result, users no longer need to rely on specific keywords or phrases, making knowledge searching and discovery more natural and effortless.
3. Knowledge consumption: Foster self-service by providing contextual and personalized responses
Self-service has always been regarded as one of the ultimate goals of successful knowledge management. By moving from the traditional method of surfing through information to a more intuitive question-and-response approach, knowledge management systems with advanced conversational capabilities can redefine the end-user experience.
When users are unable to resolve their issues because of solution articles being unclear or too lengthy, they are forced to submit a ticket to the service desk. Instead of directing the user to a bunch of knowledge articles and providing them as is, generative AI can provide an excerpt or summary of relevant articles in its response.
This enables users to quickly grasp the essence of information without having to scour through extensive content. If end users can find their answers in these generated responses, they are more likely to refrain from reaching out to the service desk.
Final thoughts
It is clear that generative AI can significantly enhance an organization’s ability to improve and share knowledge across the enterprise. From advanced content generation to automated knowledge extraction, generative AI technologies are paving the way for organizations to harness the full potential of their data.
We have yet to properly explore all the possibilities of generative AI in enterprise use cases. But there is no doubt that by seamlessly blending valuable enterprise data with the prowess of AI, we are on the brink of a new era where knowledge will be more dynamic and accessible than ever before!
About the author
